In this project, we from three groups, Augmented Speech Production group at Nagoya University (NU-G), Augmented Hearing group at Tokyo Metropolitan University (TMU-G), and Machine Learning Technology group at NTT Communication Science Laboratories (NTT-G), to conduct research on coaugmented sound media communication.
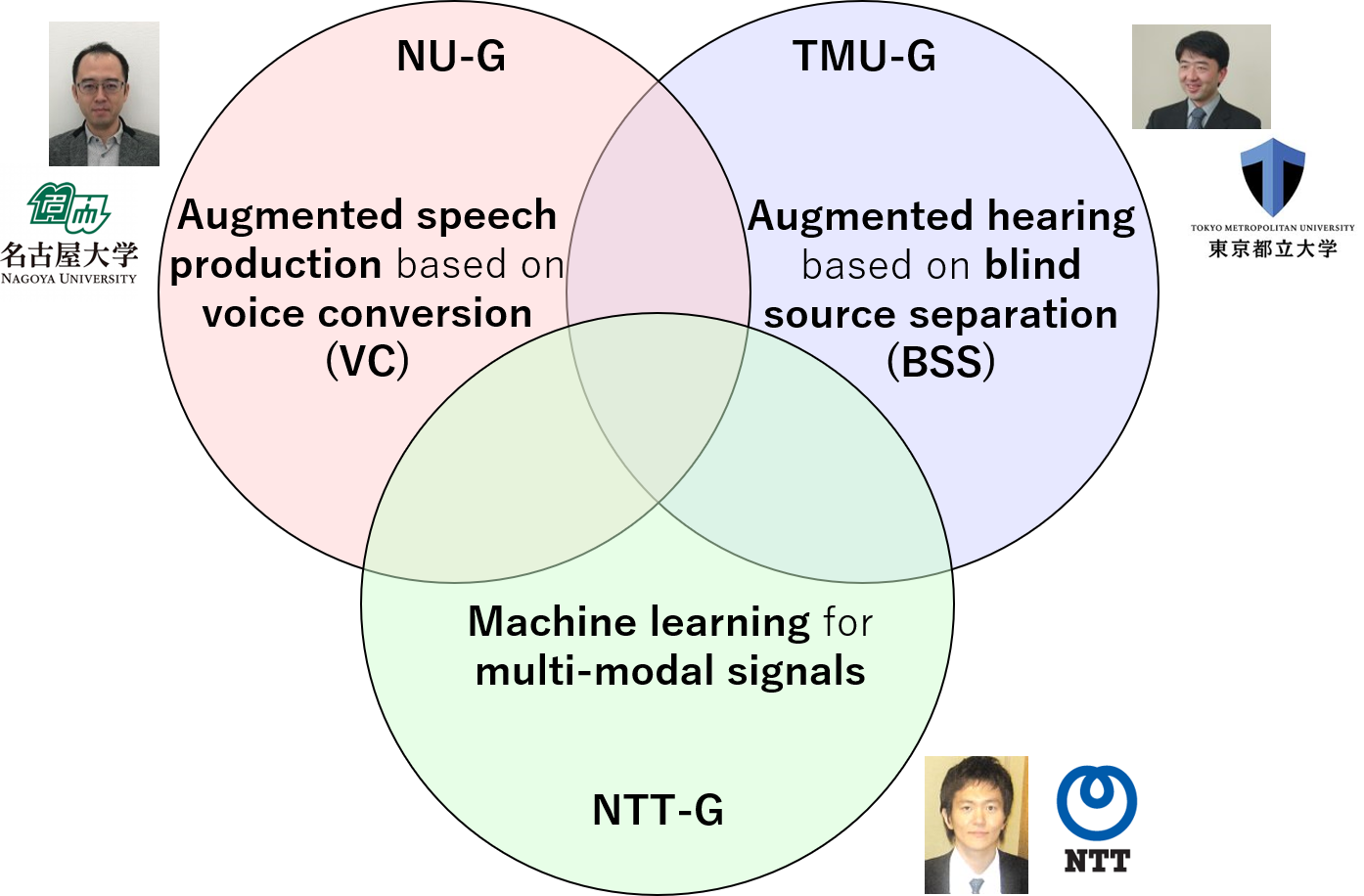
Augmented Speech Production Group (NU-G)
We will develop fundamental techniques for cooperatively augmented speech production to produce desired speech and singing voice beyond physical constraints by using low-latency real-time voice conversion (LLRT-VC) processing based on machine learning. Moreover, we will also develop speaking- and singing-aid systems for recovering disabled people’s speech production and speaking- and singing-enhancement systems for augmenting general people’s speech and singing production functions.
To use these systems as a part of our physical functions, we need to develop a framework enabling users to dynamically control speech and singing expressions of converted voices as they want. However, in some applications, e.g., speaking- and singing-aid systems for vocally handicapped people, it is challenging to estimate speech and singing expressions that they intend to produce since their produced speech suffers from a lack of these expressions due to physical constraints. To address this issue, we will establish techniques to intentionally control them by using multi-modal behavior signals as an additional input. As a final goal, we aim to develop cooperatively augmented speech production techniques effectively using user-system interactions for users to understand the system's behavior.
Augmented Hearing Group (TMU-G)
We will establish fundamental techniques for cooperatively augmented hearing to selectively listen to a target sound by using LLRT multi-channel audio signal processing based on machine learning. Moreover, we will also develop hearing-aid systems for hard-of-hearing people and hearing-enhancement systems for augmenting general people's hearing functions.
One of the essential issues in hearing aids is the difficulty of selectively amplifying only a target sound that a user wants to hear. A simple amplification process also amplifies noise, hindering the user's hearing. Blind source separation (BSS) is a technique to separate mixed sounds without using any prior information about the position and direction of sound sources and has the potential to be applied to hearing aids. However, since it is a blind process, there is a fundamental problem the user does not know which of the separated sound signals is the target sound. In addition, most of the current audio signal processing handles an audio signal in the time-frequency domain due to computational efficiency, physical interpretability, and flexibility of model design. However, since an audio signal needs to be processed frame by frame to use its frequency representations, a delay of one frame (tens to hundreds of milliseconds) is inevitable. To address these issues, we will establish LLRT-BSS techniques using user-system interactions to enable BSS to augment the hearing function cooperatively.
Machine Learning Technology Group (NTT-G)
We will establish machine learning techniques allowing users to control the system's behaviors through user-system interactions in the cooperatively augmented speech production and hearing systems.
We will develop fundamental techniques to recognize the user's intention from multi-modal behavior signals, such as manipulation actions, gaze movements, gestures, and facial expressions, and accurately control the output of data-driven systems based on the recognized user's intention. Beyond the uni-directional system control by the user, we will try to build a methodology to support that the user and the system can cooperatively learn each other's behaviors, and the system can automatically fill in the gaps in the user's senses through the use of the system. We aim to develop fundamental techniques that guarantee the system's safety to ensure the user can use them with peace of mind.